ENSO forecasting method based on deep learning
A deep learning and forecasting model technology, applied in the field of data processing technology and machine learning, can solve the problems of large ENSO forecast deviation, achieve the effect of solving large forecast deviation, improving forecast accuracy and accurate forecast
- Summary
- Abstract
- Description
- Claims
- Application Information
AI Technical Summary
Benefits of technology
Problems solved by technology
Method used
Image
Examples
Embodiment Construction
[0024] It should be noted that, in the case of no conflict, the embodiments in the present application and the features in the embodiments can be combined with each other. It should be noted that the terminology used in the present invention is only for describing specific embodiments, and is not intended to limit the exemplary embodiments according to the present application.
[0025] Such as figure 1 As shown, a deep learning-based ENSO prediction method provided in Embodiment 1 of the present invention includes:
[0026] S101: Obtain several physical element data observation sets from different ways;
[0027] S102: Process the physical element data observation set so that all physical element data observation sets have the same data format;
[0028] S103: Pre-extract several physical element fields in the physical element data observation set to obtain training features;
[0029] S104: Import the physical quantity of each element in the training feature into the model throu
PUM
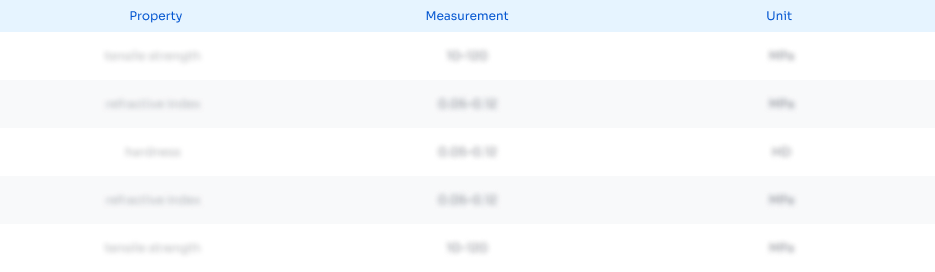
Abstract
Description
Claims
Application Information
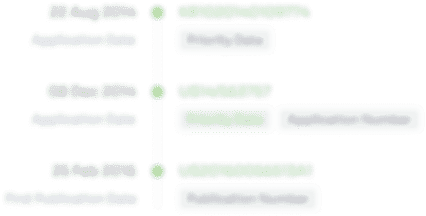
- R&D Engineer
- R&D Manager
- IP Professional
- Industry Leading Data Capabilities
- Powerful AI technology
- Patent DNA Extraction
Browse by: Latest US Patents, China's latest patents, Technical Efficacy Thesaurus, Application Domain, Technology Topic.
© 2024 PatSnap. All rights reserved.Legal|Privacy policy|Modern Slavery Act Transparency Statement|Sitemap