Comprehensive data augmentation method based on small sample medical image
A medical imaging and comprehensive technology, applied in the field of medical image processing, can solve the problems of generalization and low precision of the automatic segmentation model, and achieve the effect of strong accuracy
- Summary
- Abstract
- Description
- Claims
- Application Information
AI Technical Summary
Benefits of technology
Problems solved by technology
Method used
Image
Examples
Embodiment 1
[0065] The training and testing process of the U-Net model is as follows:
[0066] The first step is to use the data drawn by the doctor to train the model to obtain the segmentation model; the second step is to predict the model; the data processing of the segmentation model includes data loading and preprocessing. Data loading needs to convert CT values into HU values. Perform interpolation, clipping, and normalization preprocessing on the data.
[0067] Such as Figure 3-6 As shown, the model training takes the temporal lobe of the head as an example, and obtains 45 cases of head cases, with a total of 2453 CT images. 30 cases of head cases are used for training, and the rest are used for testing. The original size of CT images is 512*512. Among them, the temporal lobe part is recorded as 1, and the non-temporal lobe part is recorded as 0; the convolutional neural network model structure is as follows figure 2 shown. It can be seen that the initial input is a CT matrix w
Embodiment 2
[0074] Such as Figure 3-6 As shown, the comprehensive data augmentation methods of medical images include: rotation, scaling, three-dimensional mirror transformation, and random Gamma transformation. Rotation augmentation adjusts the main body image of the patient in the CT image clockwise and counterclockwise by randomly selecting a rotation angle in the (-15°, 15°) interval.
[0075] x rotate =Rotate(x);
[0076] Due to the difference in the size of the patient, the size of the imaging subject is very different. The data preprocessing part interpolates the data. In theory, one pixel of the image is equal to 1mm in the real world. Therefore, considering the difference in the patient's physique, the scale Scaling scales the patient subject image with (0.5,1.5) as the value interval.
[0077] x scale =Scale(x);
[0078] The purpose of mirror transformation is to solve the data differences caused by different body positions and abnormal formation of patient organs. Normally,
Embodiment 3
[0086] Based on the experimental operation of embodiment one and embodiment two, the result test obtained is as follows: the U-Net model described in this paper adopts Dice similarity as the criterion for evaluating the segmentation result, which is defined as:
[0087]
[0088] The number of cases used for testing was 15, and the test results were as follows Figure 7 As shown in the figure, the DSC results of the model trained by using the augmented data and the model trained by the original data are compared, and it is found that the test accuracy and generalization after augmentation are improved compared with the previous ones, dice Value fluctuations are more stable.
PUM
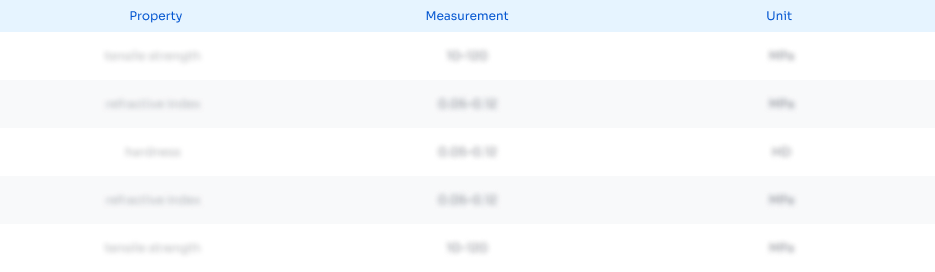
Abstract
Description
Claims
Application Information
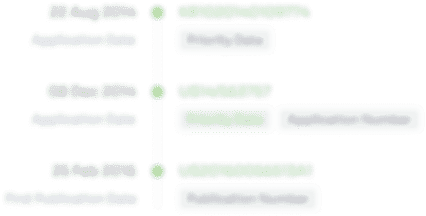
- R&D Engineer
- R&D Manager
- IP Professional
- Industry Leading Data Capabilities
- Powerful AI technology
- Patent DNA Extraction
Browse by: Latest US Patents, China's latest patents, Technical Efficacy Thesaurus, Application Domain, Technology Topic.
© 2024 PatSnap. All rights reserved.Legal|Privacy policy|Modern Slavery Act Transparency Statement|Sitemap