Retinal effusion segmentation method and system based on semi-supervised deep learning network
A deep learning network and deep learning technology, applied in the field of retinal fluid segmentation based on a semi-supervised deep learning network, can solve problems such as limited segmentation ability, low accuracy, and high cost, so as to enhance generalization ability and improve learning ability , the effect of improving accuracy
- Summary
- Abstract
- Description
- Claims
- Application Information
AI Technical Summary
Problems solved by technology
Method used
Examples
Example Embodiment
[0042] Example one
[0043] Such as figure 1 As shown, a retinal fluid segmentation method based on semi-supervision deep learning network is provided in this embodiment;
[0044] S1. Labeling the retina OCT image, obtains the label data and the non-marking data, based on the label data and the non-marking data to build a retinal OCT database, based on the retinal OCT database division training data set and test data set;
[0045] Among them, the obtained annotation data and the wound data include:
[0046] The retinal OCT picture is labeled in the pixel grade, wherein the retinal OCT picture of the pixel grade annotation is constructed as labeling data, and the retinal OCT picture of the pixel grade label is constructed to be unmarked data;
[0047] The label data in the retina Oct database is divided into training data sets and test data sets.
[0048] In this embodiment, the 2814 sheet OCT image is acquired from 141 patients with different medical institutions. Two ophthalmologists
Example Embodiment
[0098] Example 2
[0099] Such as Figure 7 As shown in the present embodiment, a retinal fluid division system based on a semi-supervision depth learning network includes: acquisition module, first build module, a second build module, and training module;
[0100] Specific, acquisition module, first build module, the second build module, and the training module are sequentially connected, the acquisition module is used to mark the retina OCT picture, obtain the label data and the non-marking data, based on the label data and the non-marking data to construct a retinal OCT The database is based on the retinal OCT database division training data set and test data set; where the acquisition module also includes a label module; the label module is used to target the retinal OCT picture, wherein the retinal OCT image of the pixel grade label is constructed as a label Data, the retinal OCT picture of the pixel grade label is constructed as a non-label data; the first build module is used t
PUM
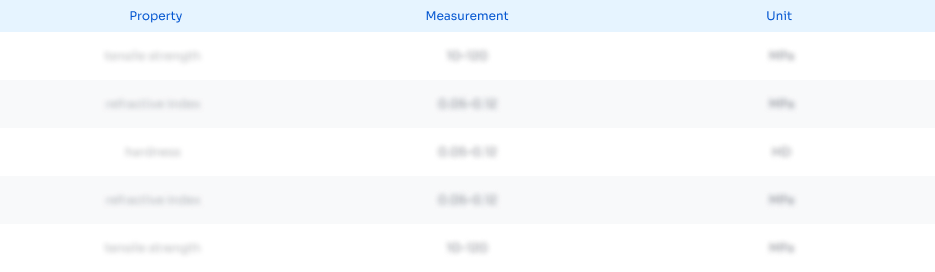
Abstract
Description
Claims
Application Information
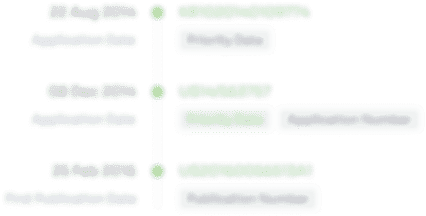
- R&D Engineer
- R&D Manager
- IP Professional
- Industry Leading Data Capabilities
- Powerful AI technology
- Patent DNA Extraction
Browse by: Latest US Patents, China's latest patents, Technical Efficacy Thesaurus, Application Domain, Technology Topic.
© 2024 PatSnap. All rights reserved.Legal|Privacy policy|Modern Slavery Act Transparency Statement|Sitemap