Main transformer fault diagnosis method based on self-adaptive reinforcement learning
A technology of self-adaptive enhancement and main transformer, which is applied in the direction of scientific instruments, instruments, measuring devices, etc., and can solve problems such as large errors, multiple fault diagnoses, and high diagnostic costs
- Summary
- Abstract
- Description
- Claims
- Application Information
AI Technical Summary
Problems solved by technology
Method used
Image
Examples
Embodiment 1
[0031] Taking the chromatographic data of the main transformer oil as an example, the AdaBoost algorithm is used for the transformer fault diagnosis problem, and 10 decision trees are trained, and then the weighted judgment is made. The input features include gases in the oil and gas spectrum, namely hydrogen (H2), methane (CH4), acetylene (C2H2), ethylene (C2H4), ethane (C2H6), carbon monoxide (CO), carbon dioxide (CO2), the amount of total hydrocarbons , and in order to take into account the advantages of the three-ratio judgment method, three ratios, namely C2H2 / C2H4, CH4 / H2 and C2H4 / C2H6 are also introduced as input features. The output fault types are divided into 10 categories, and the fault types and corresponding marking numbers are shown in the table below.
[0032]
[0033] The oil chromatographic data of 220kV, 330kV and 750kV transformers were analyzed respectively, and divided into training set and test set according to the ratio of 7 to 3. The number of sam
Embodiment 2
[0046] For transformer data with a small amount of data, if it is trained and judged according to the training results, it will face the problem that the amount of data is too small to make the training model unstable. In order to solve this problem and verify the versatility of the method, the model obtained in Example 1 was used to analyze the chromatographic data of 220kV transformer oil with a small amount of data and a different installation position from that of the sample in Example 1.
[0047] Adopt the Adaboost method gained model among the embodiment 1, to the oil chromatographic data of 95 groups of transformers, the confusion matrix of gained diagnostic result is as follows:
[0048]
[0049] According to the results of the confusion matrix, except for the normal and no-fault situation, most of the faults are concentrated in low-temperature overheating faults below 150-300 ° C, and the second is concentrated in low-energy partial discharges, which is similar to the
PUM
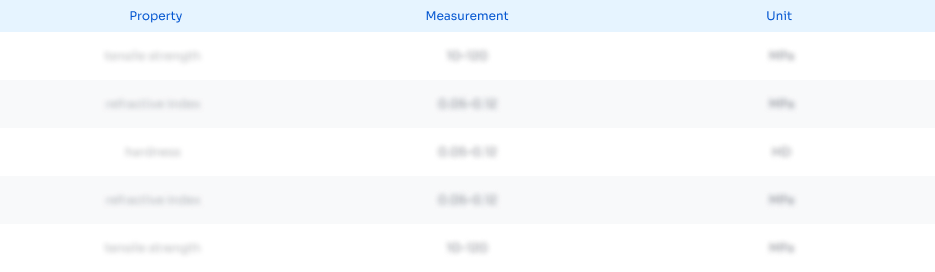
Abstract
Description
Claims
Application Information
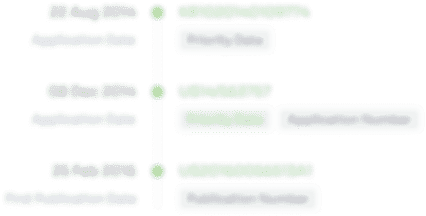
- R&D Engineer
- R&D Manager
- IP Professional
- Industry Leading Data Capabilities
- Powerful AI technology
- Patent DNA Extraction
Browse by: Latest US Patents, China's latest patents, Technical Efficacy Thesaurus, Application Domain, Technology Topic.
© 2024 PatSnap. All rights reserved.Legal|Privacy policy|Modern Slavery Act Transparency Statement|Sitemap