Multi-modal neural image feature selection method based on sample weight and low-rank constraint
A feature selection method and low-rank constraint technology, applied in the field of multimodal neuroimaging feature selection, can solve problems such as the inability to fully utilize the complementary information of multimodal data, the single feature representation method, and restrict the learning performance of the model, so as to improve the diagnosis. Accuracy, high classification performance, the effect of improving accuracy
- Summary
- Abstract
- Description
- Claims
- Application Information
AI Technical Summary
Benefits of technology
Problems solved by technology
Method used
Image
Examples
Embodiment Construction
[0031] see figure 1 , a method for feature selection based on sample weights and low-rank constraints disclosed in the present invention, comprising the following steps:
[0032] (1) Multimodal brain imaging data collection;
[0033] (2) After obtaining the data of multiple modalities, use the method of multi-modal feature collaborative analysis for feature selection, establish a regression model from each modal data to the classification class, and perform group sparse constraints on the regression vector, so as to obtain all A subset of common features that are all relevant to the task;
[0034] (3) Multimodal data feature modeling;
[0035] (4) Write the objective function as an augmented Lagrangian form, and the objective function becomes a convex function;
[0036] (5) Use the alternate direction multiplier algorithm to solve each variable in the formula in step (4) with the gradient descent method, and obtain the weight matrix W of each mode of each sample and the weight
PUM
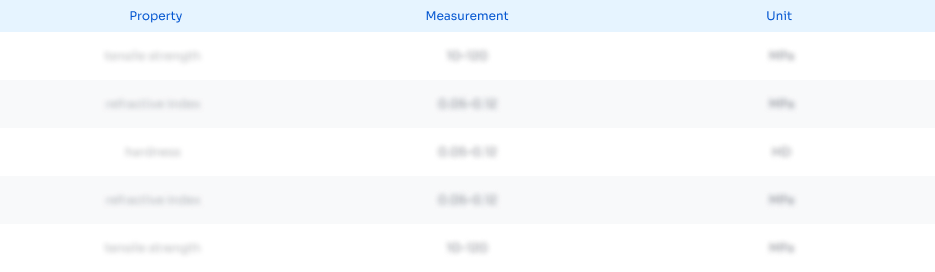
Abstract
Description
Claims
Application Information
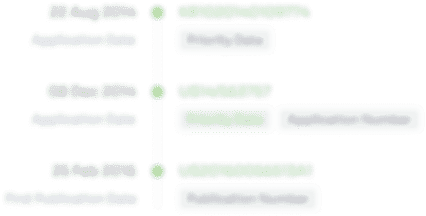
- R&D Engineer
- R&D Manager
- IP Professional
- Industry Leading Data Capabilities
- Powerful AI technology
- Patent DNA Extraction
Browse by: Latest US Patents, China's latest patents, Technical Efficacy Thesaurus, Application Domain, Technology Topic.
© 2024 PatSnap. All rights reserved.Legal|Privacy policy|Modern Slavery Act Transparency Statement|Sitemap