Dynamic width-depth neural network for hyperspectral classification and learning method thereof
A deep neural network and hyperspectral classification technology, applied in neural learning methods, biological neural network models, instruments, etc., can solve the problem of low training efficiency, improve learning accuracy, overcome catastrophic forgetting, and optimize architectural parameters.
- Summary
- Abstract
- Description
- Claims
- Application Information
AI Technical Summary
Benefits of technology
Problems solved by technology
Method used
Image
Examples
Embodiment 1
[0057] refer to figure 1 , a dynamic width-depth neural network for hyperspectral classification provided by the present invention, including P EWSWS networks in the width direction, in the learning phase, P EWSWS networks are cascaded sequentially; in the application phase, P EWSWS networks are parallel The hyperspectral data to be processed is processed; P EWSWS networks are added to the dynamic width-depth neural network one by one through the step-by-step learning process of the learning data; each EWSWS network contains L EWSWS layers cascaded sequentially in the depth direction, each Each EWSWS layer contains multiple conversion layers; each conversion layer is constructed by a width sliding window module and a subsampling module; during the sliding process, each Gaussian kernel is expanded in the depth direction.
[0058] Specifically, the construction process of the dynamic width-depth neural network is:
[0059] First, according to the data volume and classification acc
Embodiment 2
[0063] refer to image 3 and Figure 4 , the learning method of a kind of dynamic width-deep neural network provided by the invention, comprises the following steps:
[0064] For two adjacent training cycles, the residual error corresponding to the current training cycle is used as the initial expected output of the next training cycle, and the residual error of the training is gradually reduced;
[0065] For each training epoch, proceed as follows:
[0066] (1) Divide the data set to be learned into batch training subsets, and the overlap factor between two adjacent training subsets is λ, 0<λ<1;
[0067] (2) Input the first training subset into the first EWSWS network, take the corresponding data label as the expected output, update the network weights of the first EWSWS network, and obtain the first residual corresponding to the network output Error; take the first residual error as the expected output of the second EWSWS network, and at the same time input the first trainin
PUM
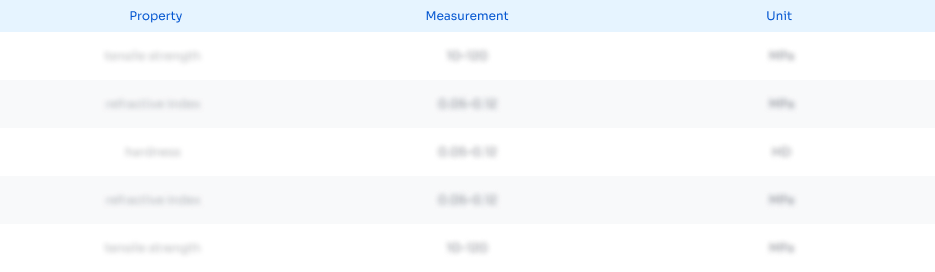
Abstract
Description
Claims
Application Information
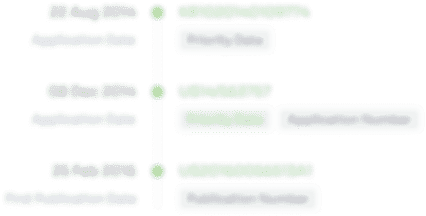
- R&D Engineer
- R&D Manager
- IP Professional
- Industry Leading Data Capabilities
- Powerful AI technology
- Patent DNA Extraction
Browse by: Latest US Patents, China's latest patents, Technical Efficacy Thesaurus, Application Domain, Technology Topic.
© 2024 PatSnap. All rights reserved.Legal|Privacy policy|Modern Slavery Act Transparency Statement|Sitemap