Detection of manipulated images
- Summary
- Abstract
- Description
- Claims
- Application Information
AI Technical Summary
Problems solved by technology
Method used
Image
Examples
Example
[0071]In a variant of the first embodiment, the BSIF feature extraction system is replaced by a single deep convolutional neural network (D-CNN) as used in the second embodiment described below—either described D-CNN may be used. It may also use the classifier system of the second embodiment, which may receive its input from the first fully connected layer of the D-CNN, as is also described in relation to the second embodiment (the feature level fusion of the second embodiment not being required).
Experiment
[0072]The inventors constructed a new large-scale morphed face database comprised of 450 morphed images generated using different combination of facial images stemming from 110 data subjects. The first step in the data collection was to capture the face images following the ICAO capture standards as defined in the eMRTD passport specification. To this extent, they first collected the frontal face images in a studio set up with uniform illumination, uniform background, neutral pose an
Example
[0085]The second embodiment of the invention will now be discussed with reference to the remaining figures. It is particularly suited to the recognition of morphed images which have undergone a print-scan process and which are therefore more difficult to detect than “digital” morphed images. The print-scan process corresponds to the passport application process that is most widely employed.
[0086]FIG. 6 shows a block diagram of system 30 of the second embodiment. As will be discussed in more detail below, it is based upon feature-level fusion of two pre-trained deep convolutional neural networks (D-CNN) 31, 32 to detect morphed face images. The neural networks employed are known for use in image recognition and are pre-trained for that purpose.
[0087]Convolutional neural networks comprise one or more convolution layers (stages), which each have a set of learnable filters (also referred to as ‘kernels’) similar to those used in the previous embodiment. The term “deep” signifies that a plu
PUM
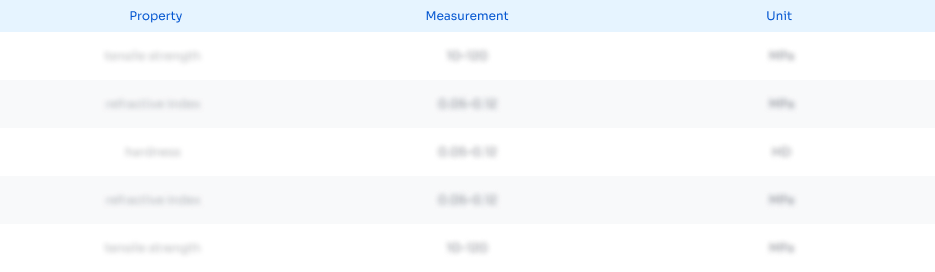
Abstract
Description
Claims
Application Information
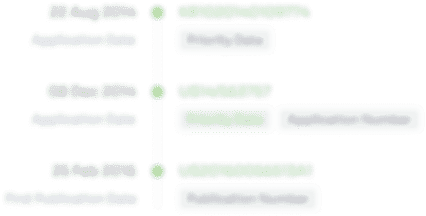
- R&D Engineer
- R&D Manager
- IP Professional
- Industry Leading Data Capabilities
- Powerful AI technology
- Patent DNA Extraction
Browse by: Latest US Patents, China's latest patents, Technical Efficacy Thesaurus, Application Domain, Technology Topic.
© 2024 PatSnap. All rights reserved.Legal|Privacy policy|Modern Slavery Act Transparency Statement|Sitemap