Medical image processing and analysis method and system, computer device and storage medium
A medical image and processing method technology, applied in the field of medical image processing, can solve problems such as high cost, influence popularization and application, and reduce the amount of sample labeling, so as to achieve the effect of reducing workload, reducing sample size, and reducing cost
- Summary
- Abstract
- Description
- Claims
- Application Information
AI Technical Summary
Problems solved by technology
Method used
Image
Examples
Embodiment 1
[0043] Embodiment 1 Medical image processing method based on deep learning
[0044] The method of this embodiment is as figure 1 shown, including the following steps:
[0045] Step 1, based on the initial labeled medical image sample training to obtain the initial deep learning model;
[0046] Step 2, input the candidate medical image samples into the deep learning model established in the previous step, complete the forward propagation process, and convert the prediction result into an entropy map based on Shannon's theorem;
[0047] Step 3: Based on the entropy map and prediction results, evaluate the total amount of information and the degree of information repetition of the candidate medical image samples, and select a batch of candidate medical image samples with the highest total non-repetitive information amount. Based on this approach, it is possible to effectively avoid the selection of samples with a large amount of absolute information but too much repetitive informa
Embodiment 2
[0063] Embodiment 2 Method and system for medical image analysis
[0064] This embodiment provides a method and system for analyzing medical images. The system of this embodiment is composed of two parts connected by data lines and data interfaces: server and computer equipment. The server is used to store data of medical images. In addition, as an equivalent alternative, the server may also be replaced by a device for collecting medical images (such as a CT machine, an ultrasound imager, etc.) or a device for inputting medical image data.
[0065] The computer device is used to analyze the medical image as follows:
[0066] The medical image to be analyzed is input into the deep learning model, and the analysis result is output after being analyzed by the deep learning model. The deep learning model is the final deep learning model obtained after training according to the method of embodiment 1.
[0067] Further illustrate the beneficial effect of the present invention in ord
experiment example 1
[0069] Dataset: The Breakhis dataset contains microscopic biopsy images of benign and malignant breast tumors, and the images are used in 3-channel RGB (red-green-blue) true color (24-bit color depth, 8 bits per color channel) color space Magnification factors 40X, 100X, 200X, 400X are obtained, corresponding to objectives 4X, 10X, 20X, 40X. The database consists of 7909 images (a total of 2480 images of benign tumors and 5429 images of malignant tumors of various scales), which are randomly divided into training set and test set according to the ratio of 3:1.
[0070] Deep learning model: The deep learning model of this experimental example is a classification model, specifically a Res-net50-based multi-scale classification network for benign and malignant breast tumor lesions.
[0071] Experimental results: According to the method of the existing technology, the full data set is used to train the deep learning model, and the classification accuracy of the trained model is 95%.
PUM
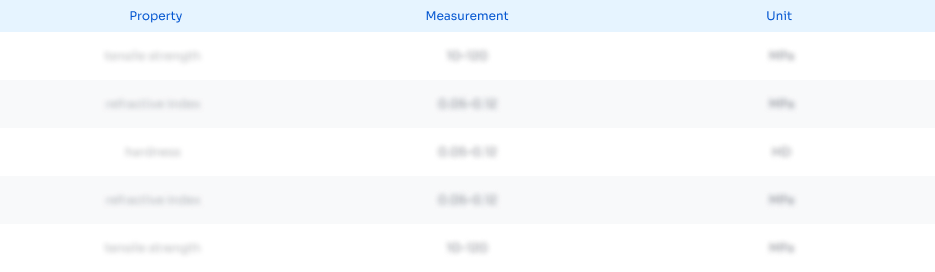
Abstract
Description
Claims
Application Information
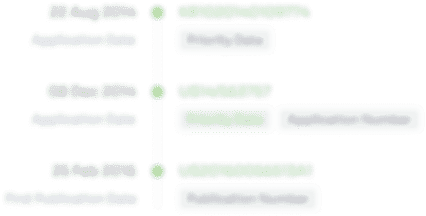
- R&D Engineer
- R&D Manager
- IP Professional
- Industry Leading Data Capabilities
- Powerful AI technology
- Patent DNA Extraction
Browse by: Latest US Patents, China's latest patents, Technical Efficacy Thesaurus, Application Domain, Technology Topic.
© 2024 PatSnap. All rights reserved.Legal|Privacy policy|Modern Slavery Act Transparency Statement|Sitemap