Multi-modal target detection method and system suitable for modal deficiency
A target detection and multi-modal technology, applied in neural learning methods, character and pattern recognition, biological neural network models, etc., can solve problems such as information loss, noise in detection results, missing modal data, etc., to improve consistency, The effect of reducing the amount of calculation and complexity
- Summary
- Abstract
- Description
- Claims
- Application Information
AI Technical Summary
Benefits of technology
Problems solved by technology
Method used
Image
Examples
Embodiment 1
[0082] Embodiment 1: A multi-modal target detection method suitable for mode loss. In the following embodiment, H is height, W is width, and D is depth. The two-dimensional RGB image data collected by the camera and the laser radar are collected. Taking the two modal data of three-dimensional space point cloud data as an example, a detailed description will be given, including the following steps:
[0083] S1. Real mode generation stage:
[0084] 1) Based on the open source multi-modal data set KITTI, obtain the 3D space point cloud data and 2D RGB image data of the lidar in the same time and space;
[0085] 2), the two-dimensional RGB image data is reflected in the mathematical expression, that is, the modal feature tensor with a size of (H, W, 3), which respectively represents the height, width and RGB three channels of this image; the Resnet network is used As a feature extraction unit of two-dimensional RGB image data, the extraction accuracy is further improved, and finally
PUM
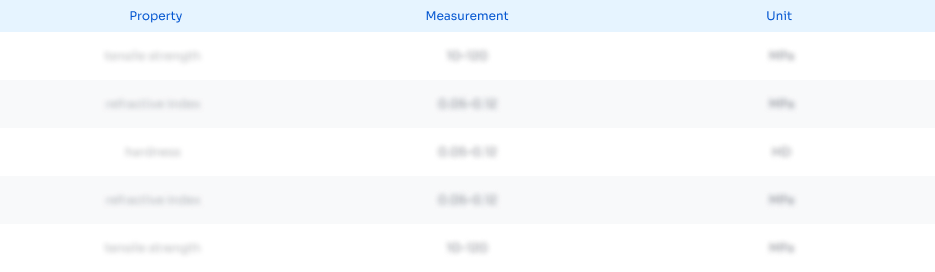
Abstract
Description
Claims
Application Information
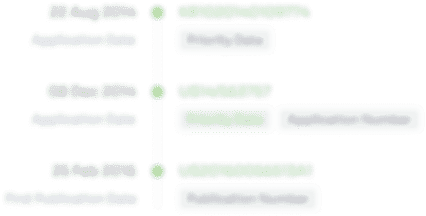
- R&D Engineer
- R&D Manager
- IP Professional
- Industry Leading Data Capabilities
- Powerful AI technology
- Patent DNA Extraction
Browse by: Latest US Patents, China's latest patents, Technical Efficacy Thesaurus, Application Domain, Technology Topic.
© 2024 PatSnap. All rights reserved.Legal|Privacy policy|Modern Slavery Act Transparency Statement|Sitemap