Method for expanding repvg structure model, image processing method and device
A structural model and image processing technology, applied in the field of extended repvgg structural model, can solve the problems of no parameter space, small parameter search space, small parameter space, etc., to improve the efficiency of image processing, reduce the amount of calculation, and increase the parameter space. Effect
- Summary
- Abstract
- Description
- Claims
- Application Information
AI Technical Summary
Problems solved by technology
Method used
Image
Examples
Embodiment 1
[0028] Embodiment 1: A method for extending the repvgg structure model, including step S3:
[0029] S3: Design a deep convolutional neural network based on a readable storage medium: such as Figure 4 As shown, on the basis of the repvgg structure, additional k convolutions are added next to each conv, and the k convolutions include conv1, conv2,...,convk to obtain an extended structure. Among them, the number of additional convolutions added by each layer of conv can be variable, and K can be a positive integer.
[0030] In the specific application of this embodiment, each convolution convk satisfies the following conditions: conv is followed by a bn layer, and convk is followed by a bn layer.
[0031] In the specific application of this embodiment, each convolution convk satisfies the following condition: the kernel size of each dimension of convk must be smaller than or equal to the kernelsize of the dimension of conv.
[0032] In the specific application of this embodime...
Embodiment 2
[0037]Embodiment 2: on the basis of embodiment 1, including steps: S5: each original conv of the trained model and the extra conv added have a bn layer, according to the weight of the bn layer, the bn layer is fused into Go to the corresponding conv weights, and remove bn to obtain a model fused with bn; S6: In the model fused with bn, for each original conv that conforms to the extended structure, first according to the algorithm of repvgg, according to the conv The number of groups, the original cross-layer connection is integrated into the conv weight as a special convolution with a specific weight, and then for all the additional convolution conv1, conv2, ..., convk of conv, refer to the idea of repvgg, put each The kernelsize and group of all dimensions of the weight of an additional convolution are expanded to an updated kernelsize and an updated group to make it consistent with the kernelsize and group of conv, and the new part of the weight is filled with 0; then th...
Embodiment 3
[0038] Embodiment 3: On the basis of Embodiment 2, an image processing method based on the method of extending the repvgg structural model as described above, includes steps S1 and S2 executed sequentially before step S3; after step S3, execute step S4; after step S6, execute step S7; wherein: S1, collect corresponding pictures according to the question; S2, mark the collected pictures; S4, input the pictures and corresponding label information into the deep convolutional neural network designed in step S3 Training, to obtain a trained model; S7, using the final model with integrated weights obtained in step S6 to perform image processing.
PUM
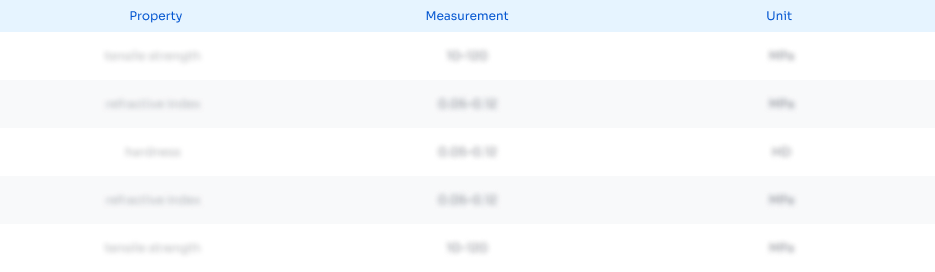
Abstract
Description
Claims
Application Information
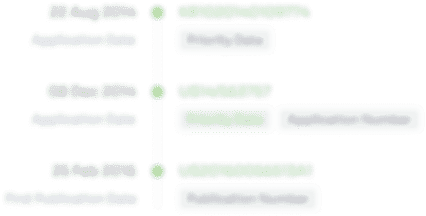
- R&D Engineer
- R&D Manager
- IP Professional
- Industry Leading Data Capabilities
- Powerful AI technology
- Patent DNA Extraction
Browse by: Latest US Patents, China's latest patents, Technical Efficacy Thesaurus, Application Domain, Technology Topic.
© 2024 PatSnap. All rights reserved.Legal|Privacy policy|Modern Slavery Act Transparency Statement|Sitemap