Long-tail distribution image data identification method based on dual-channel learning
A technology of image data and recognition method, which is applied in the field of long-tail distribution image data recognition based on dual-channel learning, to achieve the effects of improving feature representation, enhancing compactness, and improving recognition accuracy
- Summary
- Abstract
- Description
- Claims
- Application Information
AI Technical Summary
Problems solved by technology
Method used
Examples
Example Embodiment
[0032] The present invention will be further described below in conjunction with specific embodiments.
[0033] The Places365 dataset is a large image dataset covering 365 scene categories. Each category contains no more than 5000 training images, 50 verification images, and 900 test images. The original data set of Places365 is down-sampled according to the Pareto distribution with the power exponent parameter of 6, and the training set of the long tail distribution image data set contains a total of 62500 images, of which each category contains a maximum of 4980 images and a minimum of 5 Picture, the constructed training set Places-LT of the long-tail distributed image data set is as figure 1 Shown. The validation set of the long-tail distributed image data set samples 20 images of each type to track and evaluate the performance of the dual-channel learning model. The test set of the long-tailed distributed image data set samples 50 images of each type to evaluate and compare th
PUM
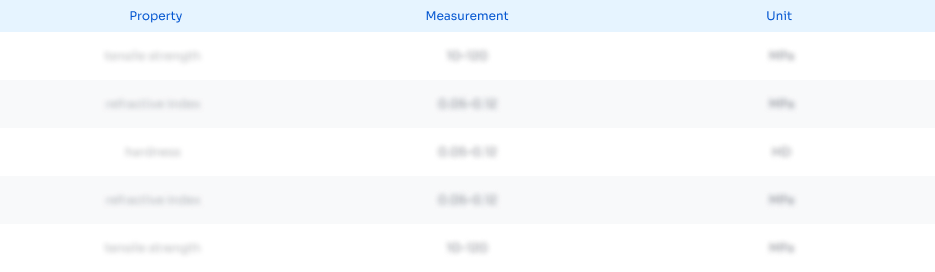
Abstract
Description
Claims
Application Information
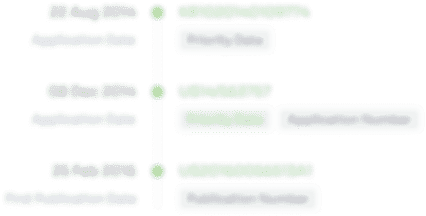
- R&D Engineer
- R&D Manager
- IP Professional
- Industry Leading Data Capabilities
- Powerful AI technology
- Patent DNA Extraction
Browse by: Latest US Patents, China's latest patents, Technical Efficacy Thesaurus, Application Domain, Technology Topic.
© 2024 PatSnap. All rights reserved.Legal|Privacy policy|Modern Slavery Act Transparency Statement|Sitemap